The French National Archives
The mission of the French National Archives is to collect, sort, describe, preserve, restore, communicate and promote public archives from the central administrations of the State, the archives of the notaries of Paris and private archives of national interest.
In order to enable their public to search and consult archival fonds, the National Archives produce finding aids (repertories, catalogues, inventories) which bring together the main metadata of files or documents: reference code/identifier, title, date, provenance, particular contents, size, volume, references to other archives or bibliographic resources, indexing of themes and names of places or persons, etc.
The conversion of handwritten finding aids
Although current finding aids are produced directly in digital form (XML EAD), the National Archives also have old finding aids, produced between the end of the 18th century and the first half of the 20th century. They are either printed, typed or handwritten. Many of them are not yet accessible online, and sometimes they are not even accessible in the reading room, even though they concern important archival fonds, particularly those of the Middle Ages and the Ancien Régime. The latter were the first to be described by archivists in the 19th century, and are therefore the ones for which the proportion of traditional finding aids is the highest.
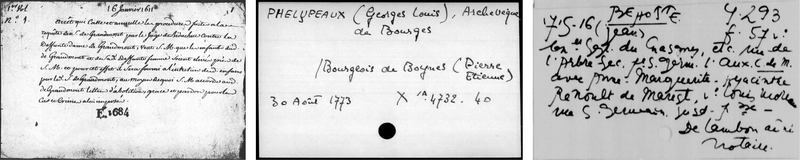
In order to accelerate the online publication of these old inventories, the National Archives chose TEKLIA to develop an application to assist in the retro-conversion of handwritten finding aids. This application relied on TEKLIA's expertise in handwriting recognition to automate a large part of the image transcription.
Reduce the burden of manual annotation production
The use of deep learning models most often requires the implementation of an annotation campaign in order to produce the annotated data necessary to train the models. In the context of the SIMARA project, the manual transcription of the records requires the help of archivists, as the reading and interpretation of their handwritten content requires palaeographic and archival skills. However, it was not feasible to mobilise archivists to produce a large amount of manual transcription. TEKLIA therefore decided not to carry out annotation campaigns, but to train the Deep Learning models directly from the result of the conversion of the records in the application by the archivists. The archivists simply used the SIMARA application to perform the conversion of records and at the same time generated training data for the models.
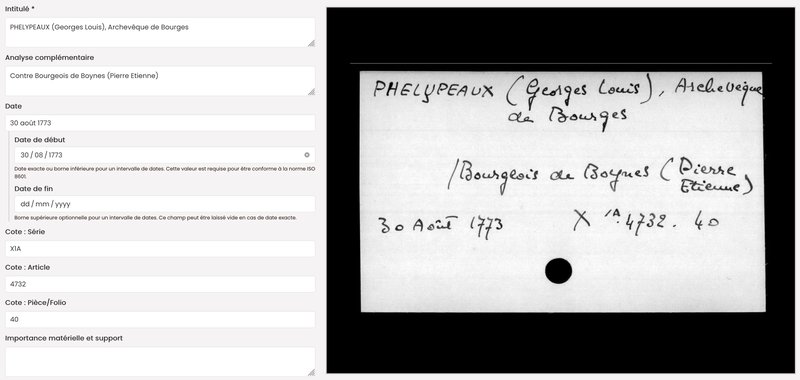
Train Deep Learning HTR with Arkindex
After TEKLIA developed the SIMARA web application, archivists started to enter information on the images of finding aids from different archival fonds. Some actions were automated in the interface, such as the normalisation of dates or the validation of entities (place names) from an XML repository.
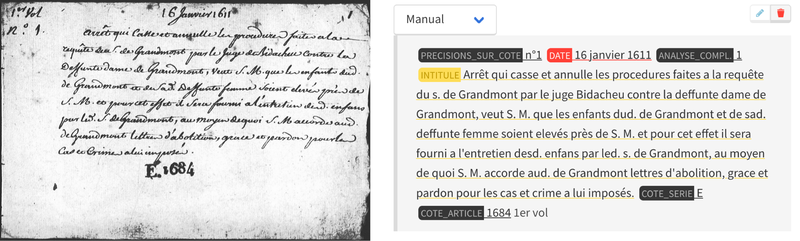
When around 200 records for each collection had been entered, TEKLIA transferred the data from the SIMARA application to Arkindex in order to train the handwriting recognition (HTR) and entity extraction (NER) models. It is important to note that the models were trained without manual annotation of the position of the information on the image, a step that usually is time consuming. Instead, the model was trained directly from the archivists' entries in the SIMARA web interface form. No specific annotation was needed to train the model.
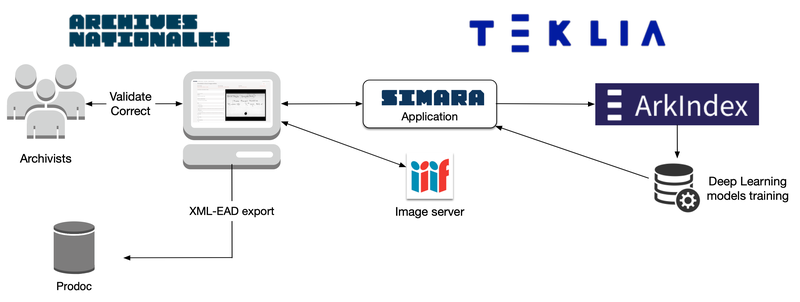
Thanks to the use of pre-training, the performances of the HTR/NER models were very good even with a small number of annotated data (880 annotated images). A character error rate (CER) of 12% was measured on an independent test set, but this value is overestimated due to differences in reading order between the annotator and the machine. In practice, archivists have been impressed by the quality of the automatic transcription. The date and reference code are recognised perfectly on almost all the cards. Abbreviations such as psse for paroisse (parish) or c.d.m. for contrat de mariage (marriage contract) are automatically expanded by the recognition model. The model was also able to handle different writing style and scanning conditions. After re-training on just under 4,000 manually validated forms, the error rate fell to 6% CER.
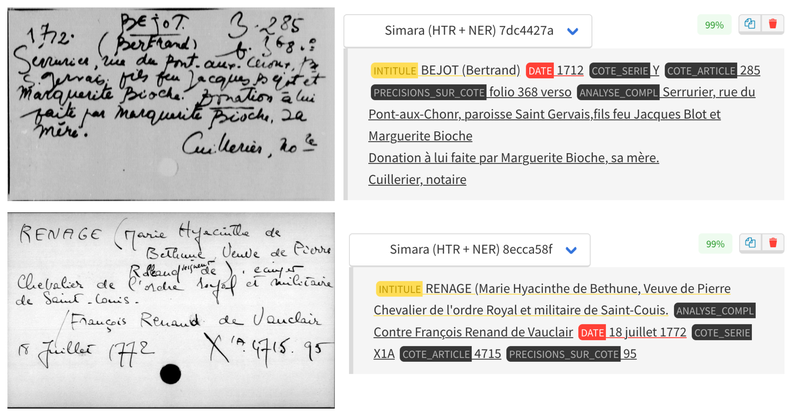
HTR-assisted data entry
The recognition/extraction model was then applied to the records not yet entered by the archivists and the result was sent to the SIMARA application as a basis for manual entry. Thanks to the automatic transcription, the archivists only need to validate and possibly correct the recognition result. The information is already placed in the corresponding form fields.
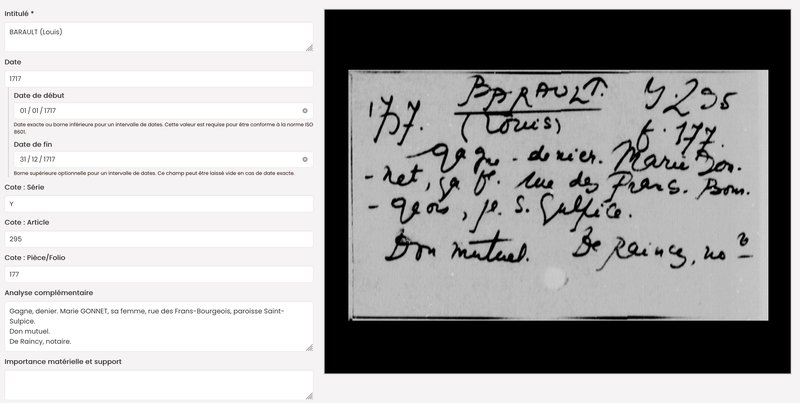
Going into production
SIMARA is now used in production at the National Archive for the conversion of the handwritten finding aids, assisted by automatic handwriting recognition and information extraction. A set of 121 finding aids, presented either in the form of registers or index cards, representing a total of approximately 100,000 pages and 800,000 cards, will eventually be converted using the SIMARA application.
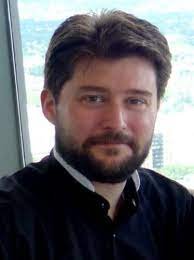
The SIMARA application represents for us a great step forward for the digital conversion of our oldest finding aids. In short, it takes advantage of AI technologies to save a great amount of time, as we are spared the most tedious tasks such as typing the whole original text or manually encoding the data into XML. These tasks were realized separately before whereas SIMARA performs them at the same time. We have very massive finding aids, such as files containing more than 100.000 records each. SIMARA helps us to handle these massive documents, as we now only focus on the data modeling and the validation of the records once they are converted. This project has been funded by the national support plan of the Prime Minister. It was considered as one of the most innovative, but this support implied a prompt development. The SIMARA interface was developed and put in production within 7 months. Even when it was not in production, we could nevertheless begin to provide TEKLIA with groundtruth data, and consequently we were delivered the automated transcriptions quite shortly after the application was released and validated by us. We are very happy of our partnership with TEKLIA. We are favorably impressed by the capacity of AI to handle difficult handwritings coming from different periods, to improve its models by aggregating our corrections and to identify the category of information to encode it in the right place. We consider that processing the archives with AI will definitely improve in the future the services we can afford to our users, as we will be able to capitalize metadata not only from the finding aids, but from the archives themselves.
The application is hosted and maintained by TEKLIA.