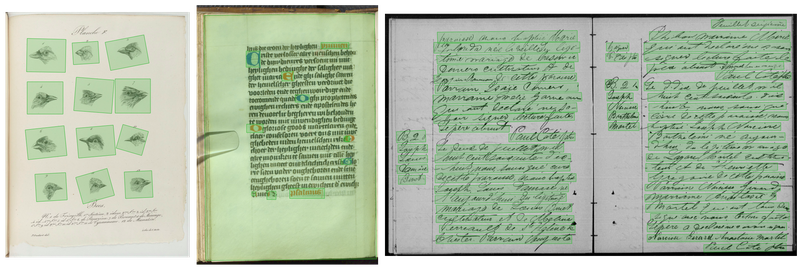
During the International Conference on Pattern Recognition (ICPR2020) held in 2021, Teklia and the LITIS (University of Rouen-Normandy) presented the Doc-UFCN model for automatic segmentation of document images in the paper Multiple Document Datasets Pre-training Improves Text Line Detection With Deep Neural Networks. This model, based on a U-Net architecture, is designed to perform different Document Layout Analysis (DLA) tasks like text line detection and page segmentation. Doc-UFCN has shown very good performances on different tasks and a reduced inference time, which is why it is now used in most of Teklia's projects.
The Doc-UFCN library is now available on Pypi and allows to apply trained models to document images. It can be used by anyone that has an already trained Doc-UFCN model and want to easily apply it to document images. With only a few lines of code, the trained model is loaded, applied to an image and the detected objects along with some visualizations are obtained.
We also provide an open-source model that detects physical pages on a document image.
Visualizations
Given an input image, the detected objects are returned as a list of confidence scores and polygons coordinates. The library can also return the raw probabilities and two visualizations: a mask of the detected objects and an overlap of the detected objects on the input image.
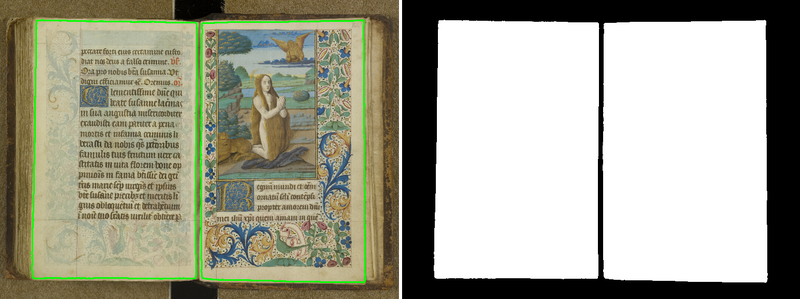